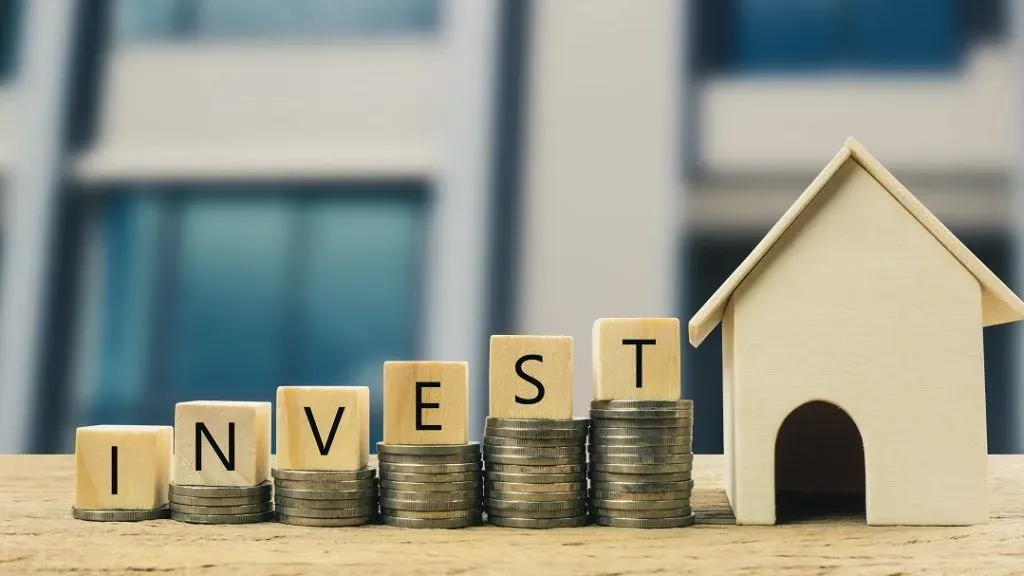
Advisors can create portfolios tailored to their clients’ financial goals and risk tolerance using advanced investment models and portfolio optimization techniques. The primary aim of these techniques is diversification by allocating assets based on expected returns and risk.
However, real world factors like regulations, taxes, and transaction costs may compromise the efficiency of optimization models.
Modern Portfolio Theory
Modern portfolio theory provides investors with a mathematical framework to select investments that provide maximum expected returns with minimal risk exposure. Diversification plays an integral role in this approach as it mitigates against individual securities’ inherent idiosyncratic risk exposures.
This approach rests on the assumption that investors are rational and risk-averse, which ignores human behaviour in financial markets. Furthermore, this methodology assumes all investors have one investment horizon without considering changes in market conditions or life events that occur over time.
Post-modern Portfolio Theory (PMPT), offers an alternative solution that addresses some of the limitations of MPT. By emphasizing downside risk and factoring in investor preferences, PMPT allows for more detailed analyses of risk vs return relationships.
Mean-Variance Optimization
The Black-Litterman Model is an adaptable form of mean-variance optimization. It combines reverse optimization with investors’ forecasts of expected returns to facilitate more active management.
The model also incorporates a correlation matrix to identify and reduce concentration in a portfolio, helping reshape its risk/return profile, which is essential to meeting investment goals.
However, this model can be limited in various ways. For instance, it assumes asset returns follow a normal distribution while neglecting non-normal behavior such as skewness and kurtosis. Furthermore, its historical data may not accurately depict future market conditions – all factors which could render Black-Litterman less useful for certain investors.
Black-Litterman Model
The Black-Litterman Model provides investors with a means of incorporating individual investment perspectives into portfolio optimization processes, allowing them to be more responsive to their own insights while remaining grounded in market realities.
Capital Asset Pricing Model combines expected market returns with investor forecasts and confidence levels for individual assets to create a recalibrating set of asset weights that aim to provide an optimal balance between risk and performance.
One key distinction from the mean variance approach is that Black-Litterman uses market equilibrium information and subjective views of investors to estimate its prior distribution parameters and covariance matrix – it’s not strictly Bayesian!
Dynamic Asset Allocation
Dynamic asset allocation refers to using a rule-based model to select an optimal mix of equity and debt assets for investments with long-term investment horizons. Funds using this approach tend to perform best.
This approach blends market data with investor perspectives, enabling it to more closely align portfolio strategies with individual risk tolerances and investment horizons while at the same time decreasing volatility for improved performance.
Equity offers the potential for wealth creation, yet can be more volatile than debt investments and challenging in times of turbulent markets. If risk aversion is something you struggle with, equity investing may not be suitable; but if you can ride out its ups and downs successfully it could yield great returns on your investment.
Multi-Objective Optimization
Numerous mathematical models and optimization techniques exist to assist traders/investors in crafting well-balanced portfolios. These advanced tools enable more informed decision making and improved risk management.
Most financial problems involve multiple objectives that conflict, such as optimizing return while simultaneously mitigating risk. Achieve one objective may hinder another, leading to an inevitable trade-off situation.
Multi-objective optimization (MOO) is an effective approach to solving these types of complex trade-off problems, using decision variables and decision tree techniques to identify a nondominated solution space within an acceptable range. Such solutions are known as Pareto optimal. MOO methods may either be deterministic (such as linear programming (LP) and quadratic programing (QP), or stochastic such as evolutionary algorithms to find optimal solutions.